And don't fear the Reaper
Fear the Black Box — Don't Fear the A.I.
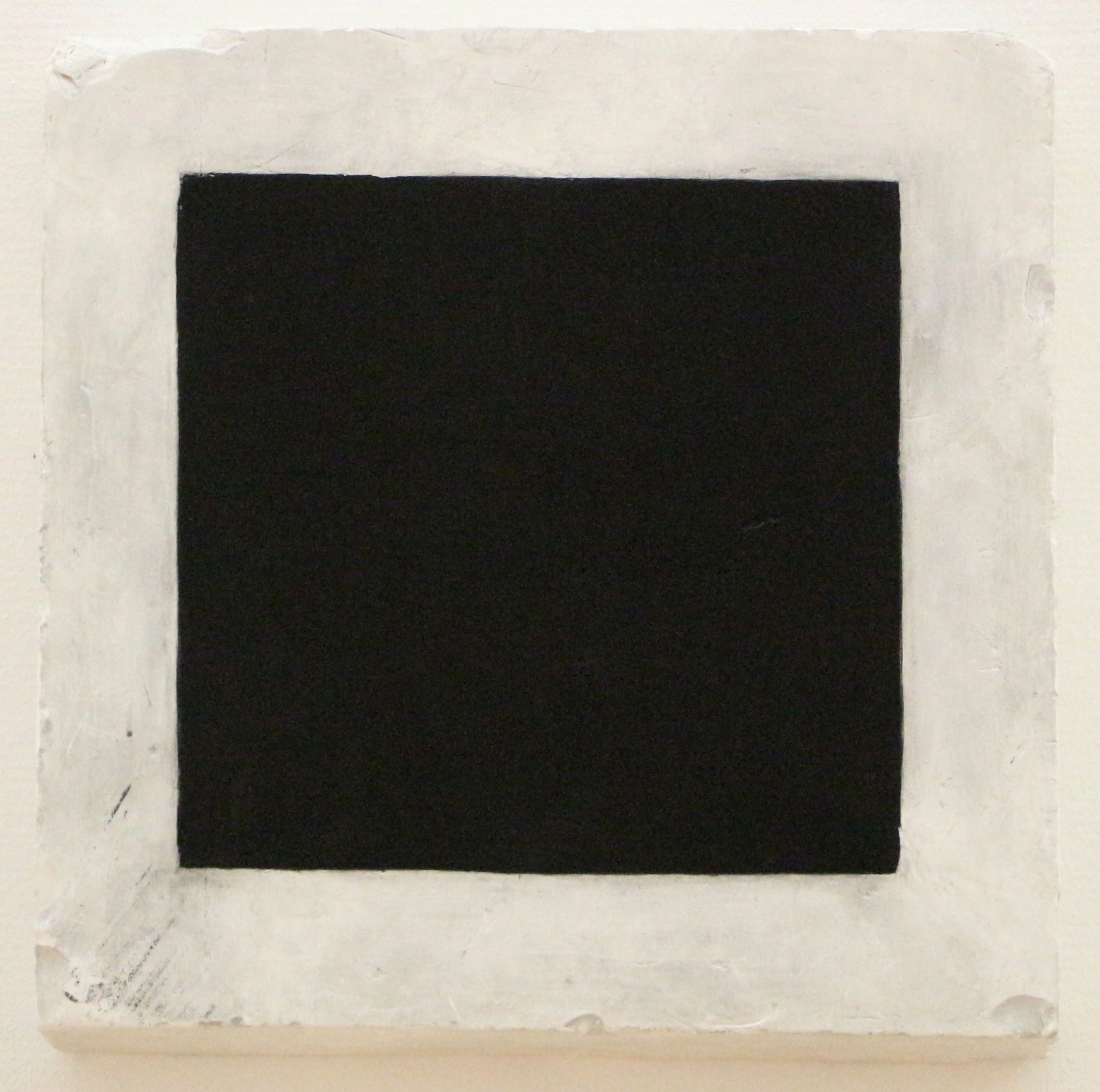
With so much public doomsaying over A.I., optimistic views on the matter make for a welcome contribution to a healthier debate on the topic. There is, however, a gap between optimism and unwarranted confidence into which Mr. Pande's recent Artificial Intelligence’s ‘Black Box’ Is Nothing to Fear seems to have fallen.
"Black box" is an engineering term describing a system where both inputs and outputs can be observed but the internal mechanics of which remain mysterious. The gist of Mr. Pande's argument is that A.I. is not more of a black box than human intelligence is, and since we trust the latter there is no reason to fear the former. Two implicit steps in this chain of reasoning are that the opacity of the inner workings of A.I is of the same nature as that of human intelligence, and that it is the sole factor with which to establish trust. Unfortunately, neither step is true.
The example of a doctor delivering a diagnosis is indeed a good one. A crucial aspect of medical diagnosis is the expectation that practitioners be able to explain themselves. Explanation does not require one to retrace the detailed order in which one's neurons fired, in fact were that possible it would likely not yield anything more than meaningless gibberish. A doctor is expected to retrace her reasoning in terms of meaningful steps that a third party could reproduce. Traceability and reproducibility are not unique to human reasoning and it is common for algorithms to exhibit these properties, but these notions directly contradict the very idea of a black box. The thought processes involved in health care are not black boxes precisely because they can be explained and reproduced. When mistakes are made, they can be corrected by pointing out at which step a wrong decision was made. Much of machine learning, however, does not afford more in the way of explanation than the software equivalent of a list of neurons firing.
The ability to provide a rationale is particularly important in the case of surprising decisions. If a doctor reaches an unexpected conclusion, she can be expected to support it with arguments that should be able to sway her colleagues. When a machine learning system produces a surprising diagnosis, it can prove difficult to know if it represents a flash of genius or a bug.
None of this argues for fearing A.I., but to the extent that it is possible we should insist on considering black box decisions unacceptable. In fact, the European General Data Protection Regulation (G.D.P.R.) which comes into effect later this year grants people the right to obtain an explanation of automated decisions that apply to them. This new law wisely requires operators of automated decision-making systems to provide their users not with the debugging tools of data scientists but rather with "meaningful information about the logic" behind the decision: one needs to open the black box through explication rather than implementation details.
Cases may nevertheless remain in which the value created by an A.I. system is considered greater than the risks associated with its inability to explain itself. Here too the example of health care can offer precedent. In medical practice it is possible to ascertain the safety and efficacy of a treatment has the intended effects without a solid understanding of its mechanism. Clinical trials are, at heart, a method of rendering black box decisions acceptably safe. As an intellectual framework there is nothing that inherently restricts clinical trials to biomedical treatments, and indeed algorithms that affect lives should be trialled no less thoroughly than drugs.
If brains and software are indeed fungible in their ability to reach decisions, then they must be equally fungible in the safeguards that surround them.