Digging into the Big Knob Theory
Competition & Privacy: It's Both Or Nothing
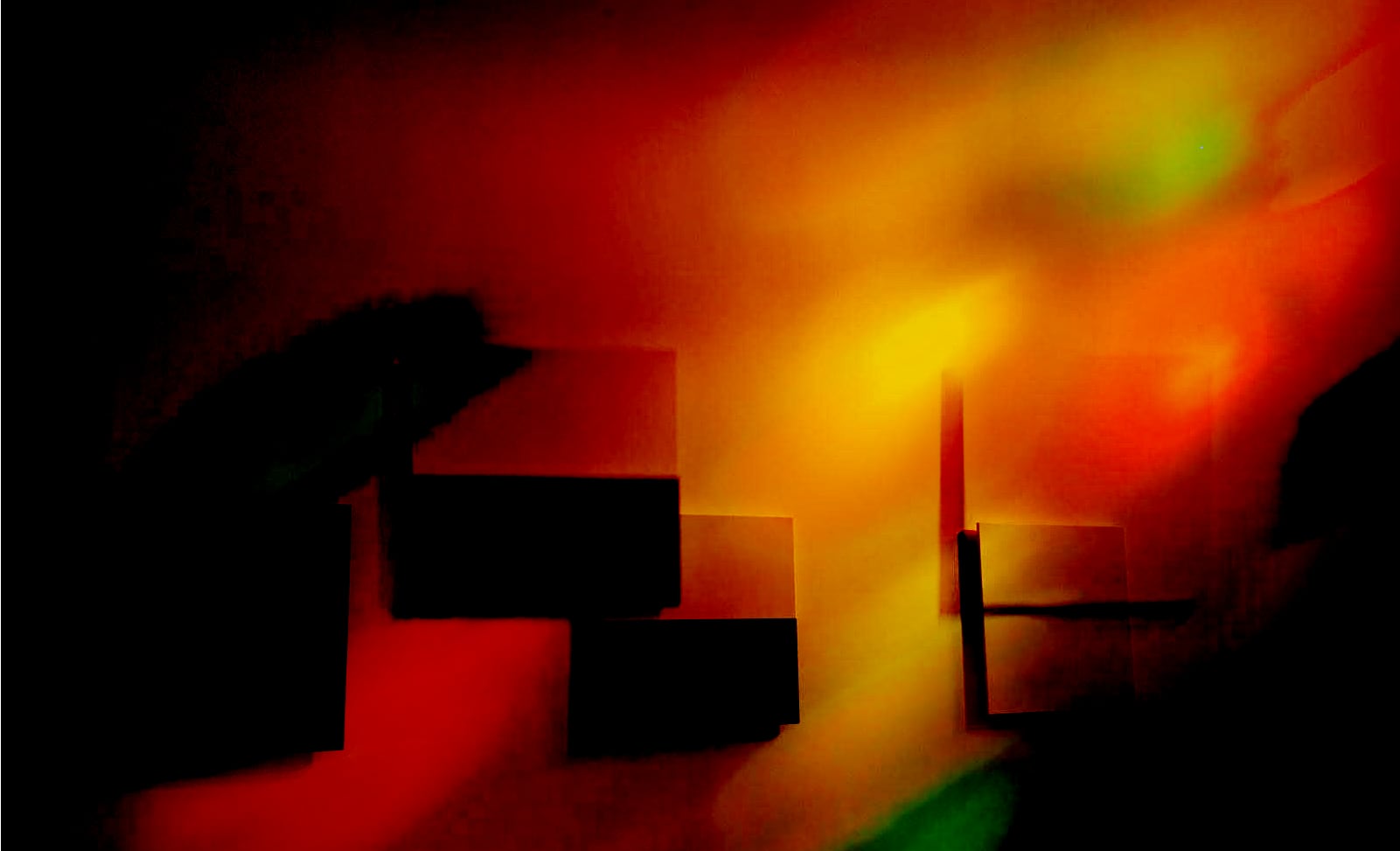
If you've spent any amount of time discussing reforms to improve privacy online, you've likely encountered the Big Knob Theory. Like Covid it comes in variants, but its core tenet can be summarised thus: there exists (metaphorically) a Big Knob that can either be turned towards "privacy" or towards "competition" — but it's very much a zero-sum game and you can't have both.
Big Knob Theory (BKT) is often a strongly-held view of its proponents, many of whom take it simply as self-evident. More surprisingly, it is also a view commonly held by those who wished things were different. You can often find them deploring the fact that they would very much like to fix our privacy predicament, but can't because it would empower companies that already have too much power.
If it's true, and certainly if as clearly true as it is taken to be, there should be solid evidence and arguments to support it. Upon closer inspection, however, it turns out that the case for the Big Knob Theory is far from being that obvious.
Let's try to formulate the situation a little more rigorously so that we have a basic framework with which to pick through the evidence.
The simplest understanding of privacy that lends itself to some degree of empirical verification is the Vegas Rule: what happens in a given context stays in that context. In practical terms, this means that whatever a person does on a given site or app cannot be learnt by a party other than that site or app in such a way that the third party can then reuse that information elsewhere. (In technical terms, the first party is the sole data controller.) On crucial points is that, under the Vegas Rule, contexts are defined as different products or services, irrespective of whether they are owned in common. Whatever happens at the Vegas Hilton will not be known at the front desk of any other Hilton, and data gathered about you by your email service will not be used by other services owned by the same company. This definition of privacy maps well to contextual integrity and to people's expectations. We can understand it as measuring the fluidity of data flows.
Competition in data or data-adjacent markets can be measured with HHI or similar metrics. (Margins can also serve as a proxy measure of how contestable the market is.)
With these starting points, an explicit formulation of the Big Knob Theory would be that data and data-adjacent markets will be more competitive in proportion to the fluidity of personal data flows between contexts, and less competitive when contexts are siloed. What support can we find for this theory?
Arguments for the Big Knob Theory
A very common argument mentioned in support of the BKT could be captured succinctly as the "Safari CPMs". The idea is that we can observe in the market that ad prices (CPMs) are measured to be significantly lower in Safari, a browser that protects privacy, than in Chrome, a browser that doesn't. But what this shows is only that if, in the same market, some parts have fluid data flows and others do not, then the money will flow to the former. Buyers who are willing to pay for decreased privacy will pay more in a system like Chrome that has a low level of security for personal data. It says nothing about the impact of data fluidity as it impacts the entire market.
The other primary argument for the BKT looks at the GDPR as a natural experiment. It comes in multiple flavours.
Some, like How GDPR is Helping Big Tech and Hurting the Competition, look at the impact of the GDPR on Google's market share. The argument proceeds as follows: the GDPR happened, but Google's market share increased anyway; therefore, privacy is bad for competition. This makes two fundamental assumptions: 1) that the GDPR improves privacy and 2) that the GDPR is being enforced against platforms. Both assumptions, unfortunately, are wrong. The GDPR, as implemented today, unfortunately includes consent as a big loophole. This has enabled pretty much everyone to broadcast personal data just as much as they did prior to the GDPR simply by adding the annoyance of consent banners. Europe has seen very little improvement in privacy from the GDPR. (And there is reason to believe that GDPR-style consent, in addition to being useless for privacy, also helps larger companies.) Additionally, the platforms are registered in Ireland, and Ireland is acting as the data equivalent of an uncooperative tax haven. Even if the GDPR improved privacy, it wouldn't apply to companies whose European operations are centred in Ireland.
Others, like Privacy & market concentration: Intended & unintended consequences of the GDPR, look at the market share of small vendors under the GDPR. Right after the GDPR comes into effect, the number of third-party vendors used in websites that operate under the GDPR drops 15% (with smaller vendors dropping more) before returning to the same level six months later (the abstract somehow fails to mention this last point). The theory here is that sites fear enforcement and so reduce their vendors — mostly the small ones that are less adept at compliance — but over time that fear of enforcement fades and the volume of third-party vendors returns.
Unfortunately, the paper doesn't factor in the realities of operating a website. Sites manage third-party vendors like this: marketers regularly want to test new vendors, and have them added to the site. When a vendor doesn't pan out, marketers are supposed to ask for its removal but that often fails (for lack of a forcing function) and stray trackers remain on the site with no purpose. When the GDPR happened (which for many was a last-minute race), pretty much every website out there had to produce a list of all its trackers, and asked marketing to explain which ones did what and who to contact to get data processing addenda in place. That's a great forcing function to spot vendors you no longer need, which alone suffices to explain the short-lived drop in the number of vendors. It also explains why the drop was short (better than the suggestion that people stopped fearing GDPR enforcement after 6 months) and why the replacement vendors are mostly different ones from those that had been removed (as noted in the paper). It's hard to overestimate the spring cleaning effect: practitioners found and shut down entire websites that should no longer have been running; a 15% drop in the number of vendors is in fact relatively small.
A great overview of this strand of thinking can be found in The Competitive Effects of the GDPR. In fact, this paper offers simultaneously a good summary of what is valuable in looking at the GDPR as a natural experiment and of why that line of inquiry does not support the Big Knob Theory. This paper (and others in this vein) tend to show that bureaucratic compliance regimes benefit large firms, as does consent-based processing. It's quite interesting to see that this kind of "notice and choice" isn't great from a competition perspective because it's also bad from a privacy standpoint.
It's worth a quick pause here because, to many, particularly outside of the privacy space, the GDPR has become synonymous with privacy. Sadly, that is hardly the case. The GDPR is first and foremost a thorough implementation of the fair information practices (FIPs), a privacy paradigm that is perfect if you are processing data in the 1970s. It is privacy that works for lawyers and compliance teams, heavily focused on procedural, bureaucratic solutions such as privacy policies, inventories, and consent. While the FIPs can be useful, and should be part of the privacy toolbox so long as their bureaucratic overhead is kept in check, there are simpler and more effective measures to improve privacy (for instance a ban on third-party data controllers) that aren't in the GDPR.
It is great that there are papers analysing the impact of the GDPR; but to the extent that they equate the GDPR with privacy, they are extending themselves beyond their empirical reach. The GDPR did not significantly and durably reduce the fluidity of data flows but it did increase the overhead of data processing in a way that favours companies with greater cover-your-ass expertise. Even if these papers do not, in fact, support the theory that improved privacy harms competition, they do strengthen the case against transparency and choice regimes.
I read a number of other papers (notably the references from those cited above) but the above points cover the spectrum of arguments that I've found in favour of the Big Knob Theory. While none actually supports the BKT itself, the related points they make can be helpful, notably in showing that certain bad ways of regulating privacy are also bad for competition.
How Privacy Improves Data Markets
In Incomplete Law, Pistor & Xu recount a history of the legal status of electricity. In the late 19th century, when the electrification of houses started to become more common, some enterprising people decided that they might as well just hook their household up straight to the grid without officially signing up for anything or paying anyone. Today, it is obvious to most that this is purely and simply theft. That view, however, was not so readily apparent to the courts. The German Supreme Court found that electricity could not be considered an asset in the sense that the law understood it, and only assets could be stolen — therefore, helping yourself to electricity couldn't be theft. American courts decidedly differently, but the matter remained contentious and was debated in New York courts until 1978.
We are facing a similar moment of confusion during which the status of data is challenging both our legal and economics traditions. Data is much weirder a commodity than electricity. It isn't easily excludable — copies are cheap and can be difficult to prevent — but it is nevertheless rivalrous (if you're the only one to know that I plan to buy expensive shoes, you can make a lot more money from shoe sellers with that information than you would if everyone knew). When traded, it becomes even weirder. The market itself is an information device. The interplay between the market as an information device with information itself being traded in that market is not straightforward.
As we increasingly apply ourselves to it, we're figuring it out. I have good hope that the 2020s will be the decade in which we begin to understand enough of digital society that we can start making it work for people.
Starting from the basics: as Neil Richards explains in Why Privacy Matters, "We live in a society in which information is power, and 'privacy' is the work we use to talk about the struggles over personal information, personal power, and personal control." Concentrations of data are concentrations of power, and from that alone we can hypothesise that those who extract the most data will wield the most power. What's more, this has the potential to create a feedback loop in which the power of data is used to capture further data, leading to greater data concentration. Just looking at first principles, privacy is the fight for more equitable and balanced power in the digital world. More equitable and more balanced power is good for competition, too. Is there any evidence for this that goes beyond basic principles?
As it happens, there is. I found good indications in the literature that the broad sharing of data across contexts has anticompetitive effects. Put differently, to the extent that there is a Big Knob somewhere, it would seem to go from "No Privacy and No Competition" to "Privacy & Competition." Given that we live in a world that is described particularly well by the former setting, this idea at least passes a smell test that the Big Knob Theory fails.
Quick interlude: since we have a catchy name for the BKT, we should also conjure one up for its inverse. Let's go with Data Accumulates Power, which Privacy Equitably Redistributes. If you're looking, it's the DAPPER theory.
Policymakers broadly tend to think that DAPPER may be true. The European Commission's Competition policy for the digital era suspects that "when it comes to dominant firms, access to more data may tend to strengthen dominance or allow an incumbent to leverage market power." The OECD's Exploring the Economics of Personal Data points out that the "monetary, economic and social value of personal data is likely to be governed by non-linear, increasing returns to scale. The value of an individual record, alone, may be very low but the value and usability of the record increases as the number of records to compare it with increases. These network effects have implications for policy because the value of the same record in a large database could be much more efficiently leveraged than the same record in a much smaller data set. This could have implications for competition and for other key policy items such as the portability of data" and their Data-Driven Innovation report had similar notes. The Stigler Center's report on Digital Platforms notes that "high and increasing returns to the use of data" tend to "push these markets towards monopolization by a single company." You can find similar indications in the CMA's Online platforms and digital advertising final report, the Furman Review, or the Cairncross Review.
Turning further towards academic sources, one great book is the classic barn-burner Big Data and Competition Policy. One perspective that Grunes & Stucke describe is the "Vs" of data valuation. Data can be seen to have some degree of relatively intrinsic value (eg. data relative to a group's willingness to pay will fetch a greater price than knowing the number of sticks in my backyard), but other important aspects also determine what can be extracted from data:
- Volume: The more data you have about one person, or the more people you have that kind of data about, the better the inferences, segmentation, etc.
- Variety: Knowing location and reading history is more valuable than the sum of the value of either taken separately, knowing the reading history from two sites is of higher value than one, etc.
- Veracity: Data the correctness of which is better established (including by obtaining it from several places) is more valuable.
- Velocity: Most of the time, the faster you know the better.
Of these aspects, volume and variety are particularly important: because the value of their sum is greater than the sum of the parts’ values, whoever has more data, especially from more varied sources, will structurally tend to outcompete whoever has less. Because of this, broad sharing of data enables increased returns to scale and scope, leading to dominance, winner-take-all, and competition for the market rather than in the market.
Another very interesting source is Competing with Big Data. The authors develop a model in which, under typical situations of broad access to cross-contextual data, data-driven markets will almost always tip. And worse, because this data is already intermixed without respect for context, it can be leveraged into other markets. (Sticking to the Vegas Rule, the power gained from surveilling people in Vegas can be used to increase one's power in Atlantic City.)
I would like to emphasise again that a clear understanding of privacy is essential to understanding the value of these models. Privacy is grounded in people-centric contexts that exist independently from whatever structures of joint corporate ownership may exist. When Google Chrome sells your data to Google Search, it isn't any less a violation of privacy than when Axciom sells your data to Facebook. The structural competition problems created by the sharing of data across contexts exist equally well in the case of tracking by third parties and in the case of internal sharing by large corporations.
Such problems of data sharing across different markets are analysed excellently in Data-driven Envelopment with Privacy-Policy Tying. Condorelli & Padilla build a simple theory in which a firm that has a dominant position in a market where data is key will use the profits from that market to enter one or more secondary data-rich markets and engage in predatory pricing there (often simply opting for free products). In turn, the data from the secondary market will bolster dominance in the primary market, leading to a vicious cycle of entrenchment. They illustrate the structure simply:

Crucially, this model only works if the firm is able (thanks to technology) and allowed (thanks to law and the practices of its privacy policy) to exploit data captured in one market in another. I find this model particularly appealing because it strongly matches the behaviour of dominant firms in the real world.
It's worth noting that in some models, data sharing can be pro-competitive, but you basically have to share all the data. Intuitively, if you nullify the advantages from data collection you eliminate competition issues in data. This works in Competing with Big Data and with the "Vs" model too: if everyone has all the data, then there are no structural advantages to volume or variety. Even if the privacy issues weren't insuperable (which they are), building the infrastructure to grant everyone access to all the world's personal data would present a huge challenge.
As we can see, there are good reasons to believe that sharing data across contexts creates competition issues — the DAPPER theory seems to hold water, and what's more to match the reality of how dominant firms behave. Is there anything that we can do about this?
Some Potential Solutions
My primary purpose here was to share my own travel through the literature to indicate that, indeed, we have solid reasons to believe that sharing data across contexts creates competition issues and that the Big Knob Theory, while we can learn from it in refusing transparency and choice frameworks, generally seems unfounded. I can, however, share a few pointers towards what I think could be solutions.
The first step is that we should pursue strong context silos whenever possible so as to avoid the structural issues that stem from cross-context sharing. One simple policy prescription is to ban third-party data controllers (with perhaps a tiny set of very narrow and strictly enforced exemptions). There is little business benefit to them and they produce great privacy harm. It is important to note that, as per the joint CMA/ICO statement Competition and data protection in digital markets (notably §79), intra-company transfers between different services should be similarly outlawed. CPRA-like regimes should treat different services as different businesses in their definition of "sale" as that more accurately reflects reality and privacy impacts.
Another important step would be to enforce strongly against self-preferential practices that create differential access to data. I think specifically of cases in which a company uses its operating system, app store, or browser to prevent others from sharing across contexts, but avails itself of data coming from interactions with these systems. This remains a problem when the effects are indirect, for instance observing behaviour on the sites of competitors in the ad market to improve search services. Increasing the scale of the latter is anticompetitive in the former. This would include enforcing The Separation of Platforms and Commerce.
Of course, building privacy-preserving alternatives (so long as they don't make it possible to exploit data dominance in other ways) to today's data-hungry services can help a lot. More generally, we can consider better ways of structuring data-adjacent markets: instead of pooling all the personal data collected from smaller sites into ever-bigger companies that then gain the ability to outcompete smaller ones, we should set the data market up in such a way that it trades in insights derived locally from the data. This enables greater innovation at the edges by empowering publishers to put their greater local knowledge of their own audiences to work and to monetise that instead of the raw material. This approach strongly aligns competition objectives with improved data protection by severely limiting the need for sharing personal data. It is a key component of several ongoing proposals to reform online advertising, such as Microsoft’s PARAKEET or The New York Times’s GARUDA. (I wrote about a high-level overview of the general idea in Identity isn’t about identifiers – it’s about people.)
In order to support strong technical privacy at the edges, where data enters the system, we should deploy a fiduciary regime for all user agents (browsers, operating systems, voice assistants, and many more). See The Fiduciary Duties of User Agents, which I hope to update soon.
The details vary, but overall the direction is simple: many of the best competition remedies that we have at our disposal in digital markets are privacy remedies. Whichever way you come at it, the future looks DAPPER.