Fixing the Web one asymmetry of power at a time
Principled Privacy
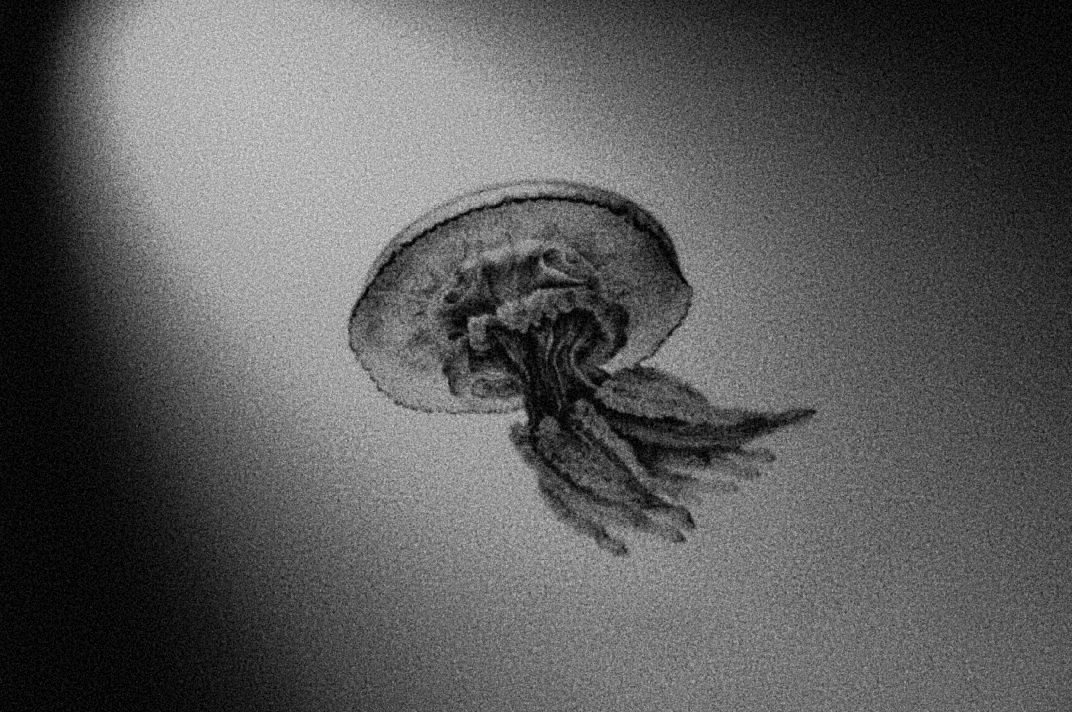
In this digital hellscape of ours, what is it that we talk about when we talk about privacy? We talk about power. Concentrations of data are concentrations of power, or, as the freshly-minted first public draft of the W3C’s Privacy Principles states, “asymmetries of information and of automation create commanding asymmetries of power.” That’s the problem to which privacy is the solution.1
When talking about the power of data, many jump straight to assuming that that power is in “AI” or in advertising (on in “the algorithm,” whatever that is). But machine learning is often weaker, at times much weaker, than claimed or hoped for, and is often only made powerful by the responsibility given to it rather than by its intrinsic capabilities. And ads are, all things considered, highly ignorable. Data does improve them, but their effective power is limited. It’s not these which I am most concerned with.
Data is power in product design. Nudging didn’t need data to be invented, but information and automation render it much more alienating. The key to understanding the alienation of nudging is to think adversarially. If you’re trying to make me do something — and for our purposes it doesn’t matter if that thing might be good or bad for me, we are only concerned with the degree of control you are exerting — there is a degree to which I may resist your nudge and a breaking point past which I can’t, or can’t without putting a lot of energy into it. For example, if you try to get me to eat more healthily by putting the healthy options at my level and the unhealthy ones higher up (a classic nudge), I can detect that. I can apply a theory of mind, or perhaps some relatively common level of cunning, and detect the trick. Or maybe I’m not that bright but you’re applying the same trick to everyone and one of my smarter friends can spot it and tell me about it. Either way, it’s well within human ability for me to go “I see what you did there, and fuck that: I’ll have me the deep-fried butter stick.”
But what happens if, instead of just designing this based on one single experiment, you are able to run thousands of experiment on millions if not billions of other people? What if you can test any number of variables on the cheap, including some really non-obvious ones like the colour, size, and shape of my cafeteria plate, the kind of music playing as I wait in line, the motif of the floor tiling? How can my more perceptive friends help me assert my autonomy if we are all getting individually-tailored nudges? And what if instead of a physical world, which takes effort to modify, I am ordering my food through a digital interface over which you have total control (in the “total institution” sense)? My ability to resist will be eroded with every single one of these changes.2
This isn’t like advertising. Advertising is mostly unrelated messages embedded in what is for the most part an ignorable form (”banner blindness” is well documented). It’s not at all the same as the ability to shape product affordances. Affordances are how we see potential in the world and how we understand our freedom of action. A door affords a handle which you see as potential to open it (whereas a bird might see it as potential to perch). The design of affordances always creates the potential for influence, but the design of data-informed affordances creates the potential for statistically irresistible control. Note that the “statistical” is important: it’s not important that under some unrealistic idealised assumptions you could resist and choose differently; what matters is that the odds are stacked against you. Such power cannot be left ungoverned.
Data is also power in mechanism design. Mechanism design is institutional engineering in which the designer picks the outcome they want from the interaction of agents and then creates rules such that agents acting freely (for some value of freely) inside the system will achieve that outcome. In a sense, it is the more general form of choice architecture and it extends to companies and entire marketplaces rather than just people.
Mechanism design can (and should) be used to create beneficial outcomes, but its neutrality is undermined by pervasive data collection and the automation it enables. A good mechanism should be understandable at all times by all participants so that they can intelligently and autonomously pick the best strategy according to their goals — but that can only work if the mechanism is “dumb.” The moment that data about participant behaviour is used to change the mechanism’s parameters in flight (often while also concealing the actions of other participants), agents lose the ability to act rationally and to cooperate with one another productively. In essence, the mechanism keeps getting modified like a maze the walls of which move any time you get closer to the exit such that agents cannot know the rules to which they are subject. This is particularly clear for instance in advertising pseudo-markets, where participants end up acting under massive uncertainty and are coordinated through mechanisms that are market-like but that lack essential features of markets (eg. freedom to deal, knowable information rules).
Such data-informed mechanisms that are “automating away markets”3 give the mechanism operators inordinate amounts of power over an entire industry or market. This too needs to be governed.
Data is, of course, power in competition settings. The Internet-wide data free-for-all is key to the process of how some firms rose to dominance. This includes access to information about other businesses’ internal operation that platform infrastructure can observe, network effects in the valuation of data that make bigger players win systematically, or the conquest of adjacent data-rich markets through predatory pricing powered by universal identifiers and lack of purpose limitations. I won’t spend much time on this, I covered it in greater detail in Competition & Privacy: It's Both Or Nothing.
These asymmetries of power need to be addressed. They threaten personal autonomy, they harm innovation and economic diversity, and they damage collective intelligence (see Stewardship of Ourselves). And this isn’t just personal data — anonymised data is often just as statistically dangerous. We can’t hope that some gentle giant will emerge that will manage all the data in a trustworthy, competent, safe way. Asymmetries of power mechanically make the powerful ignorant because power eliminates reality check, even when that power is data-driven — data isn’t knowledge, and optimising a variable based on data feedback creates control but not understanding. We learn from resistance. At the end of the day, we need a whole ecosystem of different ways of organising the world’s information — the opposite is totalitarianism whether it’s what we intend it to be or not.
It has never been easier to gently, discreetly corral people into behaviours that go against their preferences, against the choices they would have made if they were put in a position to choose freely, and more generally to impoverish their lives and interactions by standardising behaviour such as to be more legible to this or that corporation. We need to empower people to eradicate control over their behaviour. User agents cannot do everything, but they can help limit collection and rectify asymmetries of automation.
I’m under no illusion that a TAG document on its own can fix privacy for the world, but hopefully it can help align us all on a clear understanding of what privacy is for, what the stakes are, and offer principled objectives that we can all push towards.
In order to do that, the Privacy Principles are intended to learn from work at the forefront of privacy thinking4 and to render it applicable in operational, technical contexts. They also seek to provide a foundation for collaboration between legal frameworks and technical enforcement. One of the reasons that the GDPR has so far failed to deliver any systemic improvement in privacy is because it is largely unenforceable (at least not with current DPA budgets). Technology is rarely a solution on its own, but to the extent that it can eliminate reasons to collect data, enforce purpose limitation, or drive data minimisation then there is potential to render the GDPR and other regulations more operational5.
This is only the first public draft, much work remains. Please send feedback! Many thanks to my co-editor Jeffrey Yasskin and to all the participants in the task force. I’ve rarely worked with a group in which disagreements (and we have quite a few) can feel so constructive.